From Reactive to Proactive: How AI Is Redefining Cyberthreat Detection
Today’s threat environment just keeps morphing. Attackers are weaponizing AI, using advanced techniques to bypass even sophisticated defenses. Meanwhile, defenders are beginning to adopt AI-powered tools to enhance visibility, accelerate response times, and improve overall resilience.
In this blog, we explore the modern threat landscape, the role of AI in cybersecurity, real-world use cases, and key considerations when implementing AI-powered threat detection.

In the Modern Threat Landscape, Adversaries Evolve
According to CrowdStrike’s 2025 Global Threat Report:
- 79% of detections in 2024 were malware-free. Attackers increasingly “live off the land,” using built-in tools like PowerShell and RDP to avoid detection.
- Phishing attacks surged 442%. AI-driven social engineering campaigns now produce flawless emails, deepfake videos, and voice impersonations, making traditional phishing awareness harder.
- Access broker advertisements are on the rise. Attackers sell initial footholds into corporate environments on dark markets, reducing the time and skill needed for follow-on attacks.
Despite the sophistication of techniques, two initial access methods dominate:
- Exploiting stolen credentials (identity-based attacks)
- Leveraging vulnerabilities in unpatched systems
Old methods persist because they work—but the sophistication of post-breach activities has drastically increased.
How Attackers Are Using AI
Attackers are leveraging AI for:
- Advanced Phishing: Perfectly worded emails, realistic deepfake audio/video impersonations.
- Adversarial AI Attacks: Attempting to “confuse” defensive AI systems to misclassify threats.
The lines between human and machine attacks are blurring. AI doesn’t just automate attacks—it enhances creativity, scale, and believability.
How AI Defenders Are Responding
AI is transforming defensive operations across several key areas:
- Threat Detection & Anomaly Identification: AI can sift through enormous datasets across multiple systems in near real-time, surfacing threats humans might miss.
- Incident Response: Automated triage, isolation of infected endpoints, and even dynamic policy updates are increasingly AI-driven.
- Reducing Analyst Fatigue: By filtering noise and only surfacing critical issues, AI allows SOC teams to focus their attention where it matters most.
- Information Correlation: AI aggregates signals from disparate systems (EDR, NDR, SIEM, threat intelligence feeds) into coherent attack narratives.
Example: Chef Spider Campaign
A real-world APT group called Chef Spider used a novel method: submitting a malicious meeting invite through a company’s Contact Us form. The attacker persuaded an employee to download remote access malware disguised as a screen-sharing tool. Had the organization deployed AI-enhanced email filtering, EDR, and XDR systems with tight orchestration, they could have:
- Detected the malicious domain or file via threat intel correlation.
- Flagged the unusual process behavior (e.g., unsolicited remote access tool install).
- Automated endpoint isolation before the attacker escalated privileges.
This example highlights that the real power of AI lies in orchestration across multiple tools, not in a single-point solution.
Understanding the AI Engines Behind Cybersecurity Tools
It’s crucial to understand the types of AI models underpinning cybersecurity platforms:
- Supervised Learning: Trained on labeled datasets (e.g., known malware samples) for accurate detection.
- Unsupervised Learning: Finds anomalies without pre-labeled data (useful for uncovering novel attack patterns).
- Natural Language Processing (NLP): Analyzes communications (emails, chats) for signs of phishing, social engineering, or insider threats.
- Generative AI: Powers deception detection and even predictive modeling of attacker behavior.
Most effective solutions combine multiple AI techniques to balance strengths and mitigate weaknesses.
Challenges and Risks of AI in Cybersecurity
AI is powerful, but it’s not foolproof. Key risks include:
- Adversarial Manipulation: Attackers feeding false signals to confuse AI systems.
- Model Drift: Over time, without retraining, AI models can become less accurate.
- Explainability (XAI): It’s critical to know why an AI system made a decision, especially in high-stakes security scenarios.
- Over-dependence: Blindly trusting AI outputs without human oversight can lead to catastrophic mistakes.
Security leaders must prioritize solutions offering transparency, context-rich decisioning, and continuous model tuning.
Practical Recommendations for Adopting AI-Powered Threat Detection
Effective AI-powered threat detection starts with very clear steps: At a high-level, start with…
- Define Clear Use Cases. Are you aiming to reduce alert fatigue? Accelerate incident response?
- Measure Outcomes. Set quantifiable goals (e.g., reduce false positives by 30%, cut average response time by 50%).
- Prioritize Explainability. Choose platforms that offer visibility into how decisions are made.
- Maintain Human Oversight. AI augments security analysts—it doesn’t replace critical thinking.
- Address Privacy and Compliance. Understand where your data is processed, stored, and if it complies with regulatory frameworks (e.g., GDPR, HIPAA).
Conclusion: The Future of AI in Cyber Defense
AI-powered threat detection is already reshaping cybersecurity operations. It enables faster detection, richer correlation, and proactive defenses. However, it demands careful implementation, continuous tuning, and a foundational understanding of its strengths and limitations.
Security teams that embrace AI thoughtfully—as a force multiplier, not a silver bullet—will gain a decisive edge against the next generation of cyber adversaries.
Interested in exploring how AI can transform your cybersecurity operations? We offer AI readiness assessments, governance workshops, and strategic advisories to help you implement AI-powered security capabilities responsibly and effectively. Reach out to learn more.
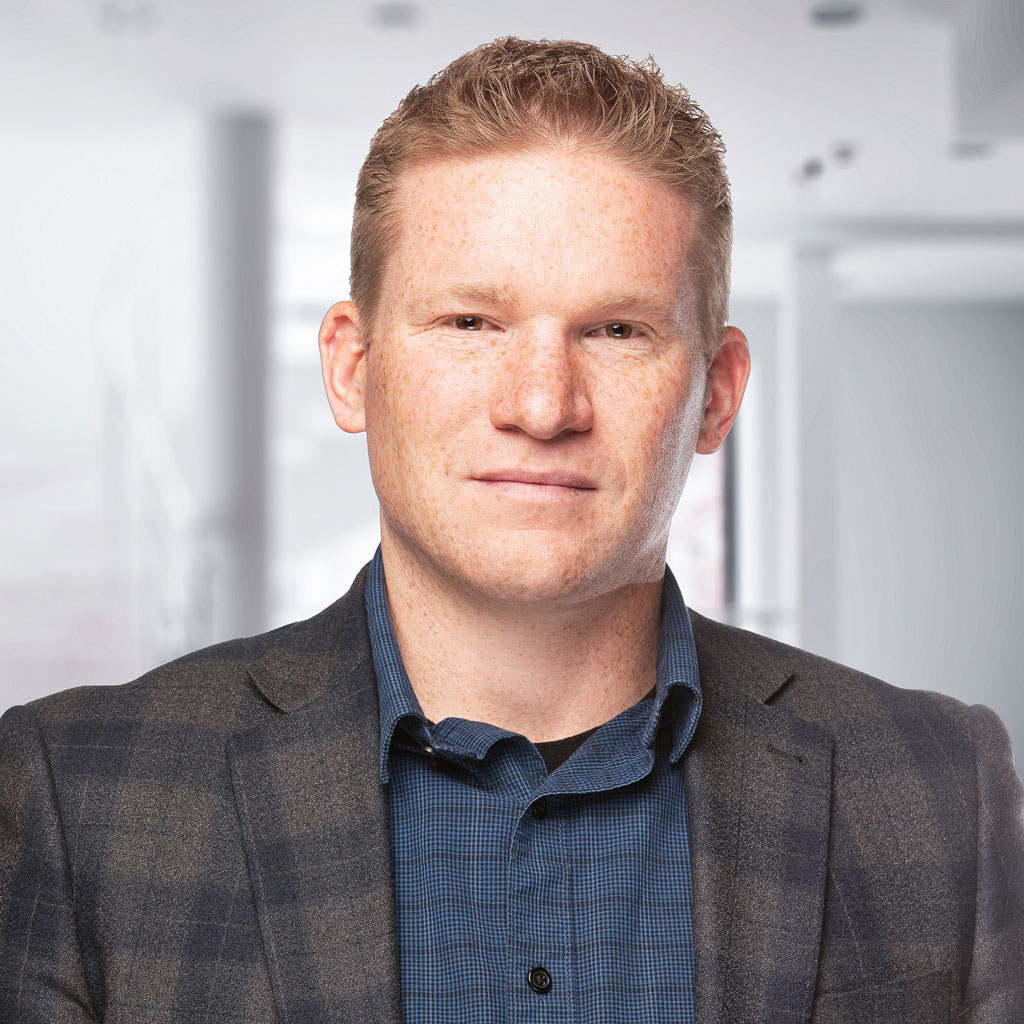
Chris Hammer
Cybersecurity Solutions Architect
Over his career, he has been responsible for conducting and supporting information assurance and cybersecurity for public utilities, financial services, state and local government, healthcare and software development companies.
Chris specializes in the design, implementation and assessment of secure infrastructure systems and integrated network applications for large, complex networks. He also focuses on processes, techniques and exploits that facilitate the development of tools and methodologies to enhance infrastructure assessments and red teaming activities.
Fortifying Digital Identity: How to Secure Access, Prevent Breaches, and Stay Compliant
In today’s digital complexity, identity security has become a cornerstone of an organization’s cybersecurity strategy. With cyberthreats growing in sophistication, IT leaders are under increasing pressure to prevent unauthorized access, mitigate credential theft,...
Exchange Server On-Prem? Get Ready for CU15 and the Future of Exchange SE
If you're still running an on-premises Exchange Server, there's some important news for you: Exchange Server 2019 CU15 is still in development, and Microsoft is making sure it's as close to code equivalent as possible to the upcoming Exchange Server Subscription...
Strategic IT Spending in 2025: Maximizing Value Amid Price Hikes
In 2025, Gartner predicts global IT spending to increase by 9.8%, reaching $5.61 trillion. However, a significant portion of this growth is attributed to rising prices for IT products and services, which means that IT leaders must adopt more strategic approaches to...